Key Outcomes
- Automated classification of 20,000 customer complaints per month
- Improved early detection of issues and trend analysis
- Enhanced sentiment analysis, issue classification, and monitoring
Client Background
Our client is a leading Banking-as-a-Service provider in the U.S., partnering with numerous fintech companies to offer deposit and credit products to millions of customers. Over the past few years, the bank has experienced rapid growth, increasing its assets to $4.2 billion. As their partner and customer base expanded, their traditional approach to managing customer complaints—manually handling an increasing volume of issues—was no longer sustainable.
Recognizing the need for a more efficient and scalable solution, they turned to Cavallo Technologies to implement a modern, AI-driven platform based on Databricks, centralizing data and automating key processes.
The Challenge
The company received customer complaints across multiple channels, including emails, service logs, and social media. Manually categorizing complaints was time-consuming, inconsistent, and inefficient, preventing the company from detecting patterns early. As the complaint volume surged, identifying urgent issues and addressing customer concerns in real time became increasingly difficult. To maintain compliance and improve customer experience, they required an automated, scalable solution.
Our Solution
Cavallo Technologies developed an advanced AI-driven complaint classification and monitoring system using Large Language Models (LLMs). This solution automatically ingests customer feedback and classifies it by sentiment, topic, and priority level, enabling rapid response and comprehensive trend analysis.
Complaint Classification Engine
- Utilizes cutting-edge LLMs for sentiment and issue categorization.
- Automatically tags complaints by topics such as billing, technical support, and account management.
Monitoring and Early Detection Dashboard
- Tracks complaint trends with visual analytics.
- Identifies anomalies and emerging patterns for early intervention.
- Provides real-time monitoring for swift issue resolution.
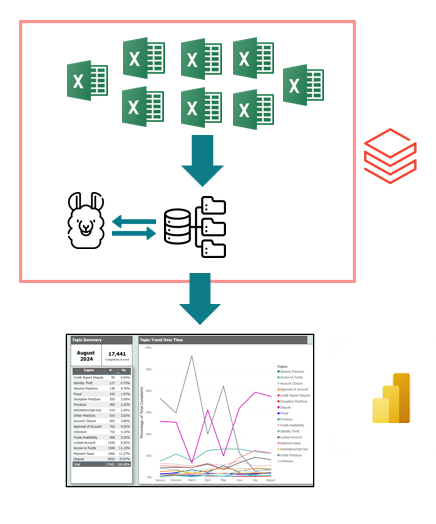
Development and Validation
To ensure accuracy and reliability, our team followed a rigorous development and testing approach:
- Data Sourcing & Annotation: Collected and structured historical complaints to train and validate the AI model.
- Prompt Optimization & Fine-Tuning: Adjusted AI models for precise sentiment detection and contextual understanding. Integrated an AI gatekeeper to prevent inaccuracies.
- Continuous Performance Monitoring: Leveraged MLflow for real-time tracking and optimization of model performance.
During deployment, the solution demonstrated remarkable efficiency, automating the classification of complaints and saving over 500 hours of manual effort annually. It achieved an impressive classification accuracy of approximately 85%, significantly enhancing operational efficiency.
Results & Impact
- 500+ hours saved annually through automated complaint classification.
- 85% classification accuracy with seamless AI integration and continuous monitoring.
- Comprehensive complaint analysis across all data, eliminating reliance on sample-based reviews.
- Improved consistency by applying standardized AI-driven complaint tagging.
- Real-time actionable insights via an interactive monitoring dashboard, enabling proactive issue resolution.
By transforming their complaint management process with AI, the fintech company enhanced compliance, improved customer satisfaction, and gained deeper insights into customer concerns, allowing them to address issues before they escalated.